Summary:
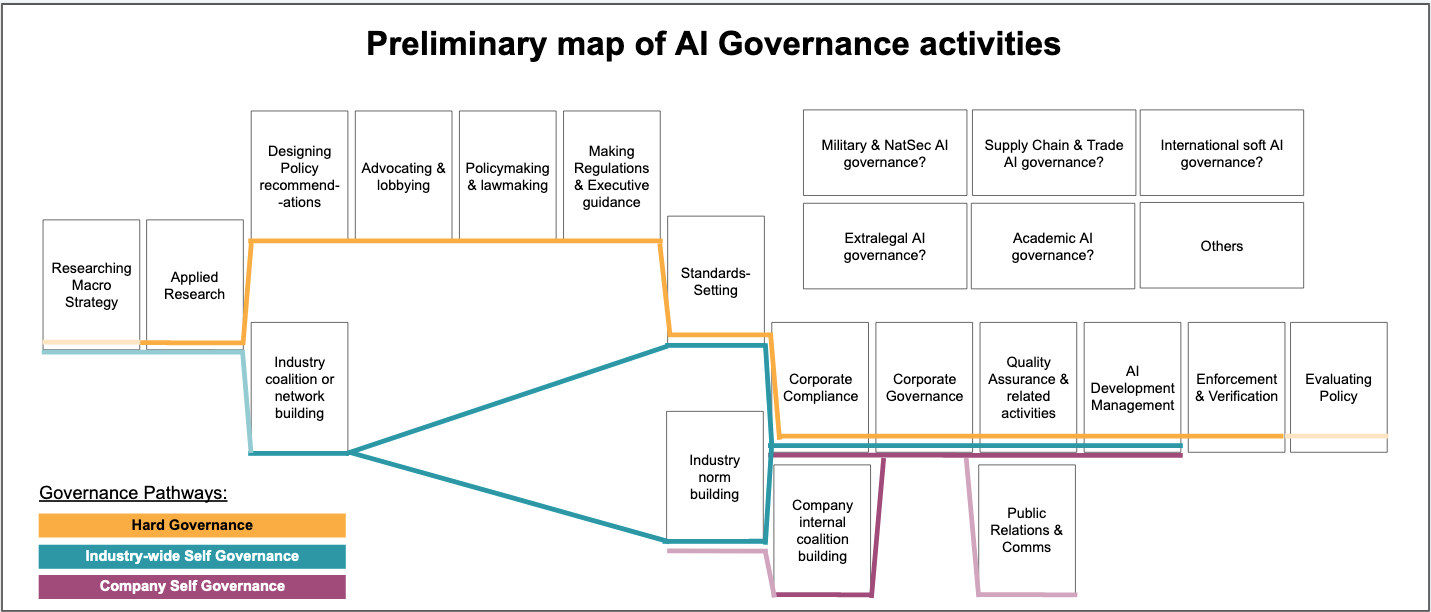
In this post, we aim to disentangle AI governance. To do so, we list and explain 17 common activities in AI governance, including typical actors, typical outputs, and examples of actions and actors underlying each activity. We describe how these activities relate to each other through three governance pathways or “macro-activities”: Hard Governance, Industry-wide Self Governance and Company Self Governance. Hard Governance refers to government’s lawmaking, which are translated into tech companies corporate policies and internal controls, which ultimately affects AI labs managers and developers. Industry-wide Self Governance refers to industry players forming a coalition to adopt new norms or standards that are then translated into companies policies, where they affect AI development. Company Self Governance relies on internal coalition-building to alter the corporate policies, which in turn affects the AI lab. Each of the three “macro-activities” is composed of some of the 17 activities we describe.
We aspire to capture all common AI governance activities, from researching macro strategy and applied research all the way to enforcement of regulations and policy evaluation. However, we identify five macro-activities for which we either have too limited knowledge or find them too specific to explain meaningfully in this overview: Military & National Security governance, Supply Chain & Trade governance, Multilateral soft governance, Extralegal governance, and Academic governance. These would benefit from further research. Finally, we list several questions for future research in the EA community.
Acknowledgments:
Thanks to Tim Fist, Aaron Gertler, Gabriella Overodder, as well as four anonymous commenters working in policy and politics, for providing feedback on various versions of this work.
How will this post be useful to you?
- You can use it to start disentangling the AI governance process and as an overview of the main activities and types of actors in the space, and of how they functionally relate to each other.
- You can “zoom in” on an activity you’re especially interested in by going straight to it using the table of content (on the left on desktop browsing) or skim several activities when you are trying to understand an actor’s function or its position in the ecosystem.
- You and others can use it as a common conceptual framework to facilitate effective actions, as well as coordination and communication.
- You can use it to enrich the assessment of your career options and to refine your own theory of change.[1]
- You can use it in conjunction with the longtermist AI governance map to identify gaps in longtermist AI governance activities or with the x-risk policy pipeline & interventions to identify some actions you can take to improve policy making.
How can you help?
This post is a first step to understand the map of the AI governance territory.[2] You can contribute to this framework by answering these three questions:
- What are the activities we have missed? How would you describe them?
- What are the nuances we have missed in describing these activities? How would you break down even further these activities?
- What other projects could help answer the research questions highlighted in the section “Further Research Questions” at the bottom of this post?
Epistemic status & other disclaimers:
- This post is based on our and our colleagues’ experience in AI governance. That experience is mostly derived from work in the EU, the US, the OECD, France, and the G7’s Global Partnership on AI, and from interactions with AI developers in the US, the UK and in multinationals.
- We hope that our map can generalize meaningfully to activities in other jurisdictions, but our explanations won’t be accurate for some jurisdictions (e.g. Advocacy & Lobbying is different in China).
- Whether we use Transformative AI, High-Level Machine Intelligence (HLMI) or related concepts makes only a minor difference in some examples we provide. The nuances (e.g. whether it’s transformative and machine-based, etc.) are irrelevant to the map in itself. We therefore use HLMI for convenience.
Governance pathways:
The activities we describe below are not carried out in isolation from each other but in more or less coordinated pipelines with supply chain-like cadence, whereby actors in one activity depend on the decisions made during activities earlier in the pipeline. It’s also possible that actors in an activity far away from AI development will directly influence AI development (for example, a software engineer may have read Superintelligence). We provide a high-level explanation of three commonly recognised pathways for the governance of AI. As we explain in a later section, there are other macro-activities (military-related AI governance, supply chain & trade-related AI governance, etc.) to further map, but these are beyond the scope of this post.
Hard Governance
Hard Governance refers to government’s lawmaking, translated in tech companies compliance and corporate policies and internal controls, which ultimately affects AI labs managers. Hard Governance operates through legally binding obligations designed by governments that arise from treaties, directives and regulations.[3] Here’s a summary of the process:
- Macro Strategy Research gives some overall direction and prioritization to AI governance applied research.
- Applied Research by stakeholders and think tanks - occasionally informed by Macro Strategy Research - generates specific insights on the governance of AI.
- These insights are further researched and turned into Policy recommendations, either by think tanks or by stakeholders with a political agenda or government agencies themselves.
- These Policy recommendations are disseminated to relevant decision-makers through Advocacy & Lobbying (either by direct outreach to lawmaking staff or by launching more grassroot campaigns leveraging citizens consultations, petitions, or protests).
- The messages from Advocacy & Lobbying are then more or less taken into account -alongside many other political, economic, social and legal factors perspectives- during the Policy and lawmaking phase. This phase results in new policies, and laws, which establish new mandates or tasks for the civil service, regulators and agencies.
- Many times, the legislative text must be further specified using Regulations & Executive guidance, which provide guidance on the policy topic. These documents translate policies into requirements for those subjected to the policies.
- Occasionally, the executive branch mandates Standard-setting organizations to further specify what “complying with the policy” technically and operationally means, prescribing as granular requirements as possible that nevertheless are still applicable industry-wide. Even if not mandated to do so, standard-setting organizations take regulations as a given and adapt their work in progress according to the new requirements, to make sure their standards are as useful as possible to as many industry players as possible.
At this point on the map, we enter the company’s internal ecosystem.
- The standards (if any) or regulatory directives are interpreted by the people in charge of Corporate Compliance. They assess the impact of various ways to transcribe these into company policies and practices. In line with the relevant mechanisms of corporate governance, they determine how to comply with the rules.
- Through Corporate governance, the leadership also adapts the company’s wider strategic decisions (e.g. dropping a product line, entering or leaving a market, investing in new testbed infrastructure, …) to maximize profits in light of the new compliance requirements.
- Individuals in charge of Quality Assurance & related activities adjust their practice based on those internal governance and compliance decisions, so as to ensure the AI development function is compliant and provides a reasonable level of quality to customers.
- AI Development Management activity is directly impacted by this adjusted practice -e.g. having to run different tests, document production differently, etc.. AI Development Management and developers’ own work and behavior adds onto the company’s compliance trace (i.e. the stream of evidence that is used to assess whether an actor complies with a rule or process, including logs, internal control systems, documentation, …).
At this point on the map, we exit the company’s internal ecosystem.
- The compliance trace is then checked e.g. by auditors, market surveillance authorities, inspectors, etc. during the **Enforcement & Verification activity. **
- Occasionally, after a few years of enforcement, the impact of the policy decisions - from communications on regulation to enforcement and verification - is evaluated during the Policy Evaluation activity. The results of this evaluation are sometimes used to make new policy recommendations.
Industry-wide Self Governance
Industry-wide Self Governance refers to industry players forming a coalition to adopt new norms or standards that are then translated into companies policies, where they affect AI development. In other words, it is the process by which firms are able to impose their normative preferences through industry-wide standards.[4]
- Industry coalition or network building is occasionally informed by Applied research, itself occasionally informed by Macro Strategy Research. Most of the time, the coalition building takes place organically in reaction to signals from the environment (e.g. mediatised backlash on lack of privacy, regulators’ increased scrutiny on unregulated aspects of production, …).
- This coalition or network, through repeated exchange of views or ideas, can sometimes find common interests strong and specific enough to propose new Standards in standard-setting bodies. Alternatively, if standardization is unlikely to be beneficial (e.g. due to concerns for politicization, attempted market predation, risk of the standard being too stringent, etc.), they aim to develop Industry norms on which a big enough share of industry could agree.
At this point on the map, we enter the company’s internal ecosystem.
- The standards (if any) or industry norms are interpreted by the people in charge of Corporate Compliance. They assess the impact of various ways to transcribe these into company policies and practices. In line with the relevant mechanisms of corporate governance, they determine how to comply with the norms.
- Through Corporate Governance, the leadership also adapts the company’s wider strategic decisions (e.g. dropping a product line, entering a new market, investing in new testbed infrastructure, …) in response to these standards, if needed.
- Individuals in charge of Quality Assurance & related activities are mandated to adjust their practice to enforce the standards and the related compliance and corporate governance decisions.
- AI Development Management activity is directly impacted by this adjusted practice -e.g. having to run different tests, document production differently, etc..
Company Self Governance
Company Self Governance relies on internal coalition-building to alter the corporate policies, which in turn affects the AI lab. It’s the company's internal process of creating or influencing the system of rules, practices, and processes by which it is directed and controlled. Corporate governance essentially involves balancing the interests of a company's many stakeholders, such as shareholders, senior management executives, employees, customers, suppliers, lenders, the government, and the community. Here is how the process works:
- A coalition within the company ecosystem (of investors or employees or other stakeholders) gathers and grows around an idea for change in the company. Note that this does not have to be vocal or politicized - internal coalition building takes place regularly on most internal matters.
- Occasionally, this idea arises from a new Industry norm that has gained momentum (e.g. the norm of giving employees the opportunity to express their opinion on whether or not to take up contracts with specific government customers).
- This coalition’s requests affect the leadership which adapts the company to it (whether constructively or not) through mechanisms of Corporate governance - e.g. a new policy, new initiative, etc. These decisions by the leadership in turn affects the company’s internal coalition strength and requests.
- The individuals in charge of Corporate Compliance and Quality Assurance and related activities then have to adapt their own processes to abide by the leadership’s decisions e.g. by setting new internal reporting requirements on computing power use, by assessing diversity among past job applicants, etc.
- In addition to this internal adjustment, the PR & Communications functions are occasionally called into action. In particular, when the internal conflict becomes significant, these functions help manage the external stakeholders’ perceptions of the company and they provide information on whether fulfilling the coalition’s requests would increase the brand value, share value or customers’ willingness to pay.
Governance pathways not detailed on the map:
- Military & National Security: refers to AI governance activities specifically related to defense actors, incl. defense contractors working on AI projects, in the context of the missions assigned to them (e.g. developers vetting; training; secrecy preservation and intelligence gathering; laws of war enforcement; development of cyberweaponry, etc.)
- Supply Chain & Trade: refers to AI governance activities specifically related to the establishment and execution of contractual agreements by and among AI-related suppliers and trade partners, and to the authorities supervising these agreements (e.g. investors arbitration courts, trade authorities, standard contractual clauses, import-export controls, …)
- Extralegal: refers to AI governance activities that take place outside the realm of the law (e.g. IP theft & code rip, cybercriminality, criminal control and trafficking of cyberweapons, commercial espionage, terrorism, etc.) and therefore in principle subject to some form of sanctions by law enforcement authorities (e.g. cyber policing, crime syndicate infiltration, etc.), and the self-governance of this extralegal domain (e.g. hackers group pacts, organized crime disciplinary measures, etc.)
- Academic and R&D: refers to AI governance activities specifically related to research, science and university-sponsored innovation activities (grantmaking, research program setting, appointments of university or AI department deans, …).
- Multilateral soft-governance instruments: refers to AI governance activities related to coordination of nation states or of actors specifically from across nation states and the norm-building that can result (e.g. UNESCO AI Principles, Asilomar Principles, G20 declarations, OECD AI Principles)
- Others: think out of the box - what are we forgetting?
Seventeen AI governance activities:
Actions can be grouped in multiple ways, and each group can be split into further activities, but at this stage of entanglement of the field, we found it useful to re-use activities labels commonly used in the policy and corporate communities, to facilitate further work with literature and entities outside of the Effective Altruism movement. This results in some ambiguous labels such as “Quality Assurance & related”, but we accept this tradeoff, supposing the description of the concept clarifies the blurry boundaries enough for now.
We indicate in parentheses our level of confidence -high or low- in our understanding of the group of activities.
1. Researching Macro Strategy (high)
Description:
This activity includes big-picture research aimed at providing an understanding of the goals of AI governance and the complicated dynamics that are bound to shape it in significant ways, as well as plans and courses of action for different actors - including people in the Effective Altruism movement - to influence AI governance.
Examples of actions:
Research on the technical landscape (capabilities, mapping, forecasting); research on foundational models of international geopolitics, domestic and mass politics, international security, notably using game theory. Research can also include imagining ideal governance, considering its most important values, principles, building positive visions of the future, institutional design and norm building. Research agendas setting up general directions and priorities for the field of AI governance are also part of this node of activities. This type of research is also carried out by grantmakers to inform their grantmaking decisions.
Types of actors:
Mostly academic centers and non-academic think tanks and funding agencies, sometimes governmental think tanks.
Examples of actors:
At the ‘whole humanity’ level: the Future of Humanity Institute at Oxford University, the Centre for the Governance of AI (GovAI), the Future of Life Institute’s through its Beneficial AGI Conference, Open Philanthropy, AI Impacts. At the EU level: the European Strategy & Policy Analysis System, the Competence Centre on Foresight of the European Commission. In the US: the Office of Net Assessment. In France: France Stratégie.
Typical or examples of output:
The book Superintelligence by Nick Bostrom, Alan Dafoe’s research agenda on AI governance, the Asilomar Principles, and forecasting reports by OpenPhilanthropy such as the Report on Semi-Informative Priors by Tom Davidson, France Stratégie’s report on governing disruptive technologies
2. Applied Research (high)
Description:
This activity explores more specific themes and topics related to the governance of AI. Rather than exploring the more global question of AI governance goals and major dynamics, they focus on one specific set of areas, such as AI & X where X is Unemployment, Immigration Policy, Climate Change, Security, etc. They can build upon the priorities laid out in the EA Macro Strategy Research activities (for example the research activities at GovAI following Alan Dafoe’s research agenda), emerge from current AI policy debates (for example, the debates in Washington DC over AI security questions inform the research at the Center for Security and Emerging Technology), or be related to AI companies internal strategic goals (for example, OpenAI Policy Research Team could conduct research on the governance of HLMI if/when HLMI is achieved by OpenAI).
Examples of actions:
Conducting surveys on Americans’ priorities regarding AI, analyzing the misuses of AI, organizing workshops at AI conferences on openness norms in the AI community, writing books touching upon AI governance, etc.
Types of actors:
Academic and policy think-tanks working on AI governance, research teams within the civil service or multilateral organizations. Some research teams in AI labs.
Examples of actors:
GovAI, FLI, the Centre for the Study of Existential Risk and its AI Initiative at Cambridge, the Center for Long-Term Cybersecurity and its AI Initiative at UC Berkeley, the Global Catastrophic Risk Institute, The Future Society, the OECD AI Policy Observatory, the Global Partnership on AI’s working groups, The World Economic Forum.
Typical or examples of output:
The report “Malicious Use of AI: Forecasting, Prevention and Mitigation”, a CSET Issue Brief providing an overview of key concepts in AI safety for policymakers, a report on using AI to tackle modern slavery, mapping AI policy initiatives, etc.
3. Designing Policy Recommendations (high)
Description:
This activity develops potential and concrete solutions to AI governance challenges applied to a specific jurisdiction, in the form of suggested decisions authorities should make about a specific topic. It also aims at setting the agenda - for example, by pushing for more safety-related questions to be discussed in AI governance fora.
Examples of actions:
Researching and writing policy briefs with recommendations to policymakers, responding to public calls for feedback on a legislative proposal, consulting companies in a specific business environment about a potential policy and writing a memo to policymakers, etc. When an individual or organization becomes a “go-to expert” for some policymakers, a common action is to answer bilateral and informal requests for input.
Types of actors:
AI companies policy teams, policy think-tanks,[5] government agencies themselves, tech companies, NGOs.
Examples of actors:
US think-tanks that focus on policy recommendations such as the Center for Security and Emerging Technology (CSET), Brookings and notably its AI and emerging technology initiative, Booz Allen Hamilton and its AI Initiative, the Wilson Center, and The Heritage Foundation. In the UK, examples are the Alan Turing Institute, the Center for Long-Term Resilience. In the EU, CEPS, Digital Europe, and Bruegel are references and the Future of Life Institute and The Future Society are also active. Note that government agencies and ministries themselves also produce a lot of policy recommendations internally. OpenAI, DeepMind and Anthropic policy teams design policy recommendations too.
Typical or examples of output:
Internal policy briefs, CSET policy recommendations for the Next Administration, the Future Proof report, response to public calls on draft guidance - for example by Google on AI policy[6] or by a coalition of academic and non-profit organizations.[7]
4. Advocating & Lobbying (high)
Description:
This activity involves actively reaching out to policymakers, decisionmakers and the public regarding AI policy recommendations. It requires deep knowledge of the inner workings of governments, including the legislative process and the people who are involved in drafting and approving such legislation. Public outreach requires skills in mass communication and/or a network of influential people, such as scientists, willing to support your cause.
Examples of actions:
One-on-one meetings with elected officials or their staff, public campaigns and use of social media, participation in policymaking workshops and forums, and advising government officials when needed.
Types of actors:
Companies’ government affairs teams, policy think-tanks external relation teams, policy think-tanks researchers briefing officials, NGOs, university lobbying teams, lobbyists and lobbying firms.
Examples of actors:
AI companies lobbying teams such as DeepMind Government Affairs team, Google corporate office in Brussels, Microsoft government affairs team and OpenAI policy team; think-tanks, universities, and nonprofits with an active lobbying or advocacy team (such as the Center for Data Innovation, the Future of Life Institute, the Centre for Long-Term Resilience, The Future Society, the Heritage Foundation, or Stanford University’s lobbying team).
Typical or examples of output:
Lobbying campaign strategies, stakeholder mapping, conferences and bilateral meetings between stakeholders and policymakers. It also encompasses actions affecting the public (e.g. open letters like the Foresight in AI Regulation Open Letter and the Slaughterbot video from the Campaign Against Lethal Autonomous Weapons).
5. Policymaking & lawmaking (high)
Description:
This activity concerns the direct drafting of laws through the legislative process. Depending on the political system in place, it can involve multiple entities and phases and the process is not always transparent. First, a law can be officially requested by the legislative assembly, by regulators themselves, or by other recognised processes (through petition, etc.). The first draft of the law is developed and proposed by whichever entity has the “right of initiative” (e.g. the European Commission in the EU), generally informed to a certain extent by the political landscape, experts and stakeholders. After that, the law is reviewed and amended by entities that have the constitutional right to do so, again informed by stakeholders if the process allows for some forms of public consultation. Finally, the final compromise text is accepted through official procedure (majority voting or other decision-making mechanisms) and it is published.
Examples of actions:
Discussing new law proposals regarding AI in intra-party and inter-party working groups, talking with lobbyists, consulting experts, building coalitions of policymakers, drafting law proposals, defending and amending them in session, voting, campaigning for becoming a policymaker, which often involves outlining some policy objectives.
Types of actors:
Members of Congress in the US and their staff,[8] members of the European Union Parliament in the EU, and representatives of the Senate or National Assembly in France, alongside their staff; policy advisors in political parties; experts in Cabinets of Commissioners/Secretaries/Ministers; civil servants in policy development units of relevant departments/ministries.
Examples of actors:
U.S. Senators Rob Portman (R-OH) and Jacky Rosen (D-NV), who led the Advancing American AI Innovation Act that was signed into law in December 2021; in the EU, Brando Benifei, Marcel Kolaja, Kateřina Konečná, Miapetra Kumpula-Natri and Alexandra Geese, who led a cross-party group of MEPs to sign a letter regarding the European AI Act; the policy development team “Unit A2” in the European Commission’s Directorate-General for Communication Networks, Content and Technology; in France, Pierre-Alain Raphan (LREM) proposed a Constitutional Law to create a Chart of AI and algorithms.
Typical or examples of output:
In the US, Congress drafted funding directives to specific areas of AI research for the National Science Foundation (NSF). In the EU, the European Commission, the Council of the EU and the European Parliament are working on the EU AI Act. In France, a Bioethics Law included precisions regarding the use of AI to manage medical data.
6. Making Regulations and Executive guidance (high)
Description:
This activity is conducted by the executive branch of governments in democracies. Regulations and executive guidance are used in three common cases. First, when there is no law giving a legislative mandate to regulators, the executive branch can publish directives, decisions, and guidance that relate to a particular aspect of society being disrupted by AI (e.g. a specific sector, public procurement guidelines, etc.). These can, for example, explain how the sectoral authority will govern AI-related innovation in its sector. Second, depending on its constitutional mandate, the executive branch can sometimes unilaterally set up programmes and initiatives that alter the way AI is being developed and deployed in its jurisdiction, such as national AI strategies or programmes for stimulating research into responsible AI. Finally, when a law gives broad requirements or ambiguous obligations, regulators and the executive branch can produce regulations and guidance explaining how they interpret and enforce the law concretely.
Examples of actions:
Consulting and communicating with various stakeholders and experts, drafting national strategies, meeting with international partners, funding research, hiring, acquiring technology, communicating and disseminating the guidance and interpretation of policy.
Types of actors:
Government, Ministries, and Government agencies.
Examples of actors:
In the US, the Office for Management and Budget, the State Department, the Department of Defense, etc. The EU has developed its Coordinated Plan on AI to stimulate AI investment across its member states. In France, the Ministry for the Economy and Finances and Ministry of Higher Education, Research and Innovation have been most involved in AI policy.
Typical or examples of output:
The White House OMB Guidance for Regulation of AI Applications, the France AI Strategy Report.
7. Standards-setting (high)
Description:
This activity relates to the development of, discussion of and agreement on standards i.e. optional requirements for the technical and governance-related characteristics of AI systems. See this footnote for more details on standards themselves.[9] Standards-setting activities bring together industry or technical experts and nation states representatives to try to reach consensus while defending their commercial interests and national competitiveness. In cases where legislation requires too much technical knowledge, government authorities sometimes mandate standards-setting organizations to develop standards “translating” the legislative requirements into technical requirements - this is the case for example with the EU AI legislation. National, regional and international standardization bodies cooperate with each other through a system of international conventions to limit geographic fragmentation of standards as much as possible.
Examples of actions:
Debating in working groups in charge of developing standards; networking with and lobbying representatives from influential national delegations; writing up sections of technical reports that feed into standards; meetings to approve or reject amendments to working “standards” documents; occasional attendance to technical demonstrations from industry players; etc.
Types of actors:
National, regional and international standards-setting organizations staff & secretariat; experts from delegations; national representatives; tech or engineering companies’ standardization, regulation, or industry development teams; industry R&D teams.
Examples of actors:
Staff and decision-makers at AI committees or working groups in ITU’s Telecommunication Standardization Sector & ISO internationally, CEN-CENELEC & ETSI in the EU; ANSI and NIST in the US; SAC in China.
Typical or examples of output:
Technical reports, which feed into standards; standard specifications, which are precursors and/or elements of full-fledged standards; testing protocols; assessment methods; definitions or production procedures to comply with in order to be considered compliant with a given standard.
8. Industry coalition or network building (high)
Description:
This activity encompasses the formal and informal convening of industry decision-makers around a common issue such as privacy or environmental impact of AI systems. The objective is generally to coordinate on principles, joint initiatives, contractual templates, etc. whose success benefits from industry coordination and benefits the coalition members individually. This can also involve representing the subject-matter interests of the industry with a common voice in public debates.
Examples of actions:
Bilateral outreach (emails, calls) to assess and drum up support for a new industry-specific institutional or infrastructural idea; organizing international forums of key AI industry players; following up and asking for introduction to relevant decision-makers; writing up and disseminating communication materials (leaflets, memos, articles, slideshow, …)
Types of actors:
Trade associations at all geographical levels and their secretariats; pre-existing supply chain or industry consortia; teams in government, civil service, civil society or international organizations in charge of stakeholder engagement or kickstarting industry alliances.
Examples of actors:
World Economic Forum; Partnership on AI; European Commission’s industry alliance initiatives (GAIA-X, Processors & Semiconductors Tech, ...); InTouchAI.eu; DigitalEurope; US Chamber of Commerce. Another national example is Hub France IA.
Typical or examples of output:
Roundtables, conferences, workshops, newsletters, members lists, interviews, manifesto, … collecting and disseminating perspectives on the issue at hand.
9. Industry norm building (low)
Description:
This activity refers to the coalitions, alliances or networks of industry players reaching a consensus on the best ways to tackle the issue at hand. In the best cases, this results in public commitments to achieving certain outcomes (such as phasing out a type of products or suppliers, rolling out new internal policies tackling the issues, harmonizing contractual practice amongst members, etc.) This activity produces more tangible outcomes than the coalition-building in section 8.
Examples of actions:
Preparing a list of recommendations that members should follow; creating a new nonprofit association hosting the secretariat for the coalition; explaining the advantages of following the new norm; discussing and adapting the norm to special circumstances of important members of the coalition; obtaining endorsements from relevant organizations (in government, civil services, academia or international organizations); setting up a public relations strategy and communication team; organizing events assessing, celebrating or reminding members of the norm agreed-upon.
Types of actors:
Significant AI industry players’ Corporate Social Responsibility (CSR), Public Relations/Government Affairs, compliance, marketing, or other relevant teams; a few outside experts endorsing the norm; staff of secretariat; individual “institutional entrepreneurs” within industry, academia, civil society or government.
Examples of actors:
Staff on the taskforce that developed Paris Call for Trust & Security in Cyberspace; Partnership on AI; World Economic Forum’s Industry 4.0 community; McKinsey & Company’s portfolio-wide Serving all Stakeholders CSR programme; Microsoft, Google, etc.
Typical or examples of output:
Set of commitments or principles endorsed by members; toolkits, series of workshops, op-ed, articles, …; new organization or institution (secretariat, committee, task force, etc.) in charge of furthering the norm.
10. Corporate Compliance (low)
Description:
This activity refers to the actions taken within each company by the staff in charge of developing and monitoring internal processes to ensure that the company respects rules it agreed to follow - whether those rules are regulations, industry standards, or softer commitments such as norms. This includes most actions by compliance teams but also throughout the company to the extent that responsibility for compliance is distributed across the organizations (e.g. product managers, Quality Assurance team, …).
Examples of actions:
Interpreting an agreed-upon rule; developing processes to respect the rule; assessing organizational and economic impact of rules and communicating it to executive decision-makers; documenting respect and infringements of the rules; training staff on compliance; monitoring and enforcing internal procedures related to external rules.
Types of actors:
Various compliance-related teams and units, either company-wide or issue- and product line- specific; compliance consulting firms; chief compliance officers; law firms.
Examples of actors:
“Security, Compliance, Privacy” units at Microsoft, the “Controls & Compliance” function at Amazon, the “Compliance, Devices & Services” team at Google.
Typical or examples of output:
Processes for respecting rules agreed upon (& implied translation of rules into standard operating procedures); training materials; reports and communication on compliance gaps and priorities; etc.
11. Company internal coalition-building (low)
Description:
This activity refers to the building up of internal political capital for establishing a new norm within the company, either by employees or investors. For employees, this build-up occurs through broadening the number of staff supporting the proposed norm or increasing the authoritativeness of supporters; for investors, it involves winning over board directors and increasing staff support. Typically, in order to establish a new way of doing things within the company, a trend or an idea needs to be captured by one or more institutional entrepreneurs who ignite the campaign around which the coalition rallies.
Examples of actions:
Outreach and assessment of relevant stakeholders attitudes vis-a-vis the suggested norm; “office politics” i.e. targeted workplace action by individuals or groups to undermine or increase the authority of some individuals on some issues; development of initiatives such as presentations at staff retreat, task forces, walkouts or strikes; discussion with leadership.
Types of actors:
Employees, investors or other internal stakeholders in more or less influential positions; stakeholders closely interacting with staff such as civil society organizations staff, union, longstanding suppliers/contractors, etc. advocating from the sidelines.
Examples of actors:
Blackrock, Statestreet & Vanguard’s directors on Big Tech Companie’’s boards; founder of the Center for Humane Technology Tristan Harris; the walkout/sit-ins/strike leaders at Big Tech Companies and their fundraisers, such as the ones related to the Project Maven or the issues surrounding Timnit Gebru departure from Google.
Typical or examples of output:
List of requests; activities to increase economic, political, social or legal pressure on the company's executive team; legal and judicial advisory.
12. Corporate Governance (high)
Description:
This refers to the actions related to decision-making at the company level or specifically related to AI. Traditional company-level corporate governance includes establishing and enforcing reporting lines and structural policies shaping the degrees of autonomy of each staff, board of directors procedures, executive team’s way of working together, etc.
AI-specific corporate governance refers to the mechanisms that AI providers have developed to govern their production of AI and related systems. Some may have created an Ethics board, an arbitration board, or a Responsible AI team. Others may have Chief AI Ethics officer-like roles, partnerships with think-tanks and academics reviewing their work, multi-stakeholder labs initiatives to facilitate oversight, etc.). Some AI companies also have dedicated AI safety teams that develop approaches to specification, robustness, and assurance for AI systems and that play a role in internal governance of AI systems development.
Here’s an example of an AI-specific corporate governance mechanism: in a given company, a Responsible AI team is tasked with managing ethical questions related to AI. It often bases its answers on AI Principles it developed within the company. The company developers or executives bring difficult or controversial situations related to AI products to the Responsible AI team, seeking counsel. For example, the issue could be about how to offset the AI system’s bias towards a minority without violating privacy of individuals in that minority. This launches a consultation process: the Responsible AI team brings together external and internal experts in law, ethics and technical AI to exchange their opinions on this issue. The experts look at the AI Principles as well as precedent cases. A decision is made that sets a new precedent, and the developers or executives are given instructions about how to deal with this situation in a responsible way.
Examples of actions:
Creation (or approval thereof) of new teams, processes, products, programmes, campaign; discussion and agreement on strategy and tactics to leverage (for example to integrate AI safety as a transversal function rather than as a silo; to get more computing power; etc.); assessing company’s possible decisions’ impact on investors; keeping executive team accountable; leading the organization.
Types of actors:
Board directors, C-suite officers, independent experts, general counsel, office of CEO staff at companies doing AI R&D, Responsible AI teams, AI safety teams.
Examples of actors:
Investors in OpenAI; Microsoft President; Google’s AI Ethics Board (defunct); Facebook’s oversight board for content management; DeepMind’s Safety & Ethics Team; general counsel of an AI company
Typical or examples of output:
Specific mandates and orders to the company staff; formal and informal sanctions and rewards based on performance’s alignment with intentions; new internal corporate policies.
13. Public Relations & Communications (high)
Description:
This activity encompasses the creation, refining, and dissemination of the company’s outgoing messages to the rest of the world as well as recommendations to leadership about what actual commitments to make in order to have more beneficial messages to send - with benefits assessed in terms of profits or liquidities, generally indirectly through the short term and long term effect on the company’s “image”. It is relevant to AI Governance as external stakeholders have at times altered companies internal policies through public criticism, which reduces the company’s image.
Examples of actions:
Analyzing and communicating the public’s attitudes towards company to leadership, crafting messages around company decisions, organizing press conferences and press releases, organizing interviews, coordinating with general counsel, suggesting actions to leadership to improve attitudes towards company, etc.
Types of actors:
In-house Press & PR and Communications teams; creatives in charge of shaping messages and image; external or consulting PR firms.
Examples of actors:
Strategic Communications, marketing function, stakeholder engagement, and some policy staff at major AI labs and companies; PR firms.
Typical or examples of output:
Multichannel communication campaigns, external communication principles and scripts, crisis response toolkit, survey of attitudes, memo with recommendations on actions to take.
14. Quality Assurance & related (low)
Description:
Quality Assurance (QA) refers to the processes and policies in place to improve, ensure and verify whether the products or services provided by the company technically meet the company’s imposed objectives and characteristics (whether self-imposed or required). We add to this group “& related” to remind the inclusion in this node of activities not typically considered as part QA, such as exploratory testing, technical documentation writing, R&D project documentation, consultations and partnership with external experts and suppliers, etc. However, at our level of granularity in activities, we fear it would be misleading to dedicate them to a separate node on the map, since many companies do not carry out these more ad hoc activities at all.
Examples of actions:
Stress testing of an AI product; testing and review of suppliers’ input (datasets, chips or pre-trained model); developing new internal testing protocols; converting international QA standards into company policies, norms and calibrations into internal processes; attending industry conferences on latest QA practices.
Types of actors:
Either a dedicated QA team/person or the responsibility is assigned directly to the product team. There can also be dedicated engineers within the company or consulting firms in QA standards compliance.
Examples of actors:
Product Safety Lead at various AI-related labs; Internal control roles at Big Tech companies; Software Quality Assurance engineers/managers.
Typical or examples of output:
Assessment of software quality and recommendations for improving quality at least costs.
15. AI Development Management (high)
Description:
This activity pools together the actions of individuals directly interacting with developers of AI systems, affecting the development of AI systems and the developers’ actions most closely. Depending on the lab set-up and organizational structure, it can include a wide range of actors. These are the actors in the ecosystem directly surrounding AI developers of whom “governance” aims to align the behavior (e.g. greater use of more inclusive datasets, greater assurance that the system will not turn everyone into paper clips during testing, …).
Examples of actions:
Translating a mandate for product development into a team’s scope of work; performance reviews; coordinating with other teams; Quality Assurance procedure enforcement; preparing ML pipeline; writing up a paper; reporting results to hierarchy; internal communications.
Types of actors:
Roles fulfilling the function of line managers, product managers, operations officers, HR managers, engineers, scientists, lab managers, …
Examples of actors:
Person in charge People’s Operations at an AI company, DevOps Ninja at a startup, ML team leader in a Big Tech organization or an AI organization, Head of Operations in a private AI lab.
Typical or examples of output:
Conversations, team meetings, establishing scope of work for a new AI system project, timelines, feedback to a teammate and formal performance assessment, team culture, level of lab cohesion.
16. Enforcement & Verification (high)
Description:
This activity includes all actions outside AI labs that serve to assess whether the actual behavior of AI developers match the mandated behavior. After a public policy has been enacted, the regulatory agency checks that the policy has been enforced among regulated entities. Two main categories of tools are reporting requirements applying to all regulated entities and controls of a sample of regulated entities. This activity thus refers to the development, deployment and operation of legal, administrative and technical mechanisms to check that a given regulation, policy and directive is complied with by actors captured by the rule’s scope. This encompasses a broad range of activities for gathering information about actors’ actions (reporting, documenting, ad hoc or scheduled controls), checking that there is no divergence from the rule (testing, auditing, certification & permit attributions, etc.) and overall policing of the industry (monitoring, analysis of reports, task force on new issues or opportunities, …). It also encompasses the development of more efficient or effective mechanisms (e.g. a software probe automatically detecting directly whether an AI system is compliant with some technical requirements rather than human examination; turning an industry standard into a requirement; ...) and the adjudication in court over legal disputes related to specific laws, which in some jurisdictions set precedents that then influence the governance of AI itself.
Examples of actions:
Assessing whether an AI lab follows existing regulations on safety in R&D; warning a provider that is not maintaining a required internal accountability level; training industry on existing policies and regulations and best practices to be compliant; negotiating with the government for obtaining a greater budget, etc.
Types of actors:
Government agencies, ministry/government department’s enforcement units, production/pre-deployment regulatory agencies, market/post-deployment regulatory agencies.
Examples of actors:
Data Protection Authorities such as France’s National Commission on Freedoms and ICT, Market Surveillance Authorities, transnational networks of regulators, Conformity Assessment Bodies.
Typical or examples of output:
Development of new governance methods; fines, red flags, warnings, investigations, sweeps, confiscation, lawsuits; formal or reasoned decisions on safety of AI development activities to e.g. launch an investigation or trigger a fine; informal decisions to e.g. contact a disgruntled AI lab employee to discuss safety of AI development activities; …
17. Evaluating Policy (low)
Description:
This activity refers to the assessment of the policy’s impact on society or industry after it has been rolled out, notably using methods of program evaluation and policy analysis. Policy evaluation can be carried out by academics as contribution to the research field or by governance institutions to inform current policymaking. Policy evaluation therefore creates a direct loop - either through “Applied Research” or “Policy recommendations”. The theoretical purpose of policy evaluation is to ensure that policies are aligned with the government expectations in terms of impact achieved, costs, or secondary effects. In practice, policy evaluation in some jurisdictions is an extremely politicized tool to undermine or strengthen some actors or to justify re-orienting policies towards new objectives of the ruling government, with little regards to the actual effectiveness. Jurisdictions differ widely in the extent to which they partake in this activity: some jurisdictions don’t evaluate any policies at all, while others legally bind governance institutions to carry out periodic evaluations of their or other institutions’ policies.
Examples of actions:
Responding to a public tender for policy evaluation, interviewing individuals relevant to the enforcement of the policy, collecting documentation from regulated entities and regulators, addressing political concerns about the evaluation underway.
Types of actors:
Specialized private research firms, think-tanks, governmental offices in charge of evaluating government activities, dedicated teams within ministries, etc.
Examples of actors:
Center for European Policy Studies (CEPS), RAND Corporation, Center for Strategy and Evaluation Services, US Office of Management and Budget, the European Commission's regulatory fitness and performance programme (REFIT), and the European Ombudsman.
Typical or examples of output:
Reports assessing some aspects of policies; policy-relevant datasets; recommendations for future adjustments to policies; reduction or increase of public budgets dedicated to specific policies, fines.
Further Research Directions:
- Further disentanglement: mapping in greater detail the activities currently left aside from the map (military, supply chain & trade, etc. …)
- Gap analysis: what activities are likely impactful but most neglected by the EA community?
- Impact planning: Which activities should more EAs be recommended to focus their careers on?
- Coordination problem: how can the EA community better coordinate across activities and pathways to ensure that there are enough incentives in the ecosystem towards HLMI safety?
- Getting closer to the territory: an interesting project for local EA groups interested in AI governance is mapping their countries’ actors involved in these activities.
Thank you for your reading - feel free to comment below with your feedback!
- ^
For a discussion on personal’s theory of change, see Lynette Bye’s article: https://lynettebye.com/blog/2020/11/16/theory-of-change-as-a-hypothesis
- ^
For a definition of these concepts, see https://en.wikipedia.org/wiki/Map%E2%80%93territory_relation
- ^
Based on two definitions: one from Martino Maggetti in his chapter “Hard and Soft Governance” in Research Methods in European Union Studies, 2015 and one from Kenneth Abott and Duncan Snidal in their 2000 article on Hard and Soft Law in International Governance in International Organization, 54(3), 421–456.
- ^
Based on the definition by Sebastian v. Engelhardt and Stephen M. Maurer in their paper “Industry Self-Governance and National Security: On the Private Control of Dual Use Technologies”, 2012.
- ^
For more information, see this EA forum post on the importance of working at a (DC) policy think-tank.
- ^
- ^
See an example of a submission to the OMB here: https://www.regulations.gov/document/OMB-2020-0003-0081
- ^
See this EA Forum post on the importance of working in Congress.
- ^
Standards are technical prescriptions of processes, outcomes or levels of performance on some dimensions that standards-setters have agreed upon as acceptable or desirable. They can refer to products, services, operational or technical procedures, etc. They are not law, regulations or executive directives. Abiding to standards is voluntary -they are not enforced by courts of law- but some standards are still widely complied with because they affect commercial opportunities: B2B supply or public procurement contracts consider bids that fulfill specific standards, regulators sometimes consider certification of compliance with a certain standard as sufficient evidence of compliance with a legal requirements, some standards are commercial symbols of technical or operational excellence, etc. Some standards are very influential on industry, others are not - this depends on the level of consensus built around the standard and the evolution of the industrial or technological landscape.
Thanks for this post!
I imagine you/readers might also find my (much less extensive and much more slapdash) mapping of The x-risk policy pipeline & interventions for improving it interesting. It's a short shortform, so I'll copy it in its entirety below for convenience:
"I just had a call with someone who's thinking about how to improve the existential risk research community's ability to cause useful policies to be implemented well. This made me realise I'd be keen to see a diagram of the "pipeline" from research to implementation of good policies, showing various intervention options and which steps of the pipeline they help with. I decided to quickly whip such a diagram up after the call, forcing myself to spend no more than 30 mins on it. Here's the result.
(This is of course imperfect in oodles of ways, probably overlaps with and ignores a bunch of existing work on policymaking*, presents things as more one-way and simplistic than they really are, etc. But maybe it'll be somewhat interesting/useful to some people.)
(If the images are too small for you, you can open each in a new tab.)
Feel free to ask me to explain anything that seems unclear. I could also probably give you an editable copy if you'd find that useful.
*One of many examples of the relevant stuff I haven't myself read is CSER's report on Pathways to Linking Science and Policy in the Field of Global Risk."
This is great, thanks for sharing this! I had not come across your comment before -not sure if Caroline did- but it's quite reassuring that despite two different approaches, two objectives, two different authorship, the overlapping descriptive part of the "steps in the chain" match almost exactly. I will edit the post to link to this.
Thank you! This is really helpful in clarifying the governance 'pipeline' and thinking about where my own works fits into this. This comment is on what others activities and actors could be included, specially journalists, media organisations and other 'cultural' influencers and activities. This comment is based on skimming the piece and on my own intuitions rather than digging too deeply into the evidence base around the role of media and cultural institutions in shaping policy and governance, so might have already considered them and decided there was insufficient evidence to include them.
The map seems to underplay the role of media and cultural actors acting with their own agency, rather than as being influenced by civil society, corporations and government. I can see why they might not be included as their role feels almost one step back from the activities of advocacy, company internal coalition-building, and public relations & communications as you’ve identified them. But their role does seem worth at least nodding to and exploring further.
For example, the section on public relations & comms talks about PR firms, creatives, in-house comms teams but these all seem like industry actors operating on behalf of the AI companies. But another side of the information environment these teams are trying to influence will be media organisations. These organisations will be influenced by those corporate teams but at the same time have their own agendas and leverage their own influence to shape policy outcomes, e.g. Rupert Murdoch’s influence in politics across multiple countries. Investigative reporting by journalists also seems to have the ability to influence opinion among the public and elites, and set the agenda for policymakers.
At another level further back in influencing change, it seems like artists, documentary makers, musicians, popular writers etc. are able to raise the salience of issues among the public and elites through their cultural work. They can both create narratives that might be more intuitive and compelling to non-experts about the importance of an issue and in using their pre-existing popularity to make an issue more visible to their followers. For example, Al Gore’s 2006 documentary An Inconvenient Truth on climate change or the activism by footballer Marcus Rashford on free schools meals in the UK during the pandemic. This feels like an even more indirect route to influencing governance and I’m unsure how impactful it might be in pushing things in a particular direction when it comes to AI, but cultural actors like Asimov, Iain M Banks or (as cliché as it is) the Terminator have seemingly influenced how the public, developers and policymakers view AI, it does seem like another possible omission from the map.
I’m not sure about the magnitude of the interventions by these actors, and whether some of these actions just folded into existing activities, particularly journalists into advocacy, but it does seem at least worth exploring the influence these actors can have.
Thanks Elliot - these are good points. In addition to the popular culture-shaping activities (by media actors and others) that you mention (i), I would add
ii) education activities (forming the future AI developers and other relevant individuals - either through the education system or extra-curricular),
iii) profession-shaping activities (carried out e.g. a professional association of AI developers), and
iv) ideology-shaping activities (e.g. by religious actors).
We decided against including them as I had not witnessed significant influence from these actors on AI governance and therefore didn't feel confident about explaining this influence (yes, to a certain extent the Cambridge Analytica scandal or France Haugen leaks have been talked about a lot, but they only shifted the mindset of relevant actors on AI because advocates/lobbyists/thinktanks/advisors kept using these scandals as excuse for altering a policy stance. For many policymakers, the scandal was resumed as "facebook = bad company", not "bad AI".) One additional source of complexity is that, given their indirect impact, it is difficult to ensure they result in a net-positive impact. For example, Unsafe at Any Speed triggered the American consumer movement but also triggered the Powell Memorandum which arguably resulted in a capture of US policymaking by for-profit interests even to date. However, uncertainty doesn't mean these activities shouldn't be considered for impact - especially since they are quite versatile (It's plausible a skilled journalist could write impactful pieces about AI, pandemics, animal welfare, effective philanthopy and global development throughout her.his career)
Likewise, we faced the question of whether to include EA community-building and grant-making activities as a meta activities influencing AI governance, but that again stretched from "traditional" non-EA definitions of AI governance.
Thanks for this! It felt a bit clarifying to me to try to group the 17 AI governance activities discussed in this post and to think about how they relate to the activities discussed in the Clarke post you mentioned. I tentatively think it’s useful to see the activities discussed in this post as a set of (non-exhaustive nor disjoint) sub-activities within the broader activities described in Clarke’s post, in this way:
Strategy research and tactics research:
Policy development:
Policy advocacy:
Policy implementation:
From this angle, a few activities seem like potential additions:
Thanks for this! Added them as examples of actions. Could you explain how "setting of corporate policy" is different from corporate compliance & corporate governance? I also didn't add the last one because it seems quite rare that policymakers would do advocacy towards corporate actors for these corporate actors to change their policy, while I believe all other activities are fairly common.
Thanks!
Re: setting of corporate policy, yeah, on second thought I'm less confident it's a very useful category. The differences I had in mind were:
Hm, maybe. My impression is that (at least in a US context and for high-profile cases) it's not so rare, since Congress members occasionally directly contact businesses or pressure them (via implicit threats of harsh legislation), by, e.g., grilling them in Congressional hearings. And advocacy from non-governmental third parties (e.g., corporate campaigns from animal advocacy organizations) might not be all that rare? But I agree it's less common than the other, standard activities.
Thanks - I see what you mean re advocacy towards corporate actors - that would make it one of the actions in industry norms building. However, I originally had congressional grillings in mind as part of the law-making process: it serves both as expressing discontent vis-a-vis companies' behavior, but also as a way to inform/signal for policymakers among themselves on the need for better policy/enforcement of the policy and on the prioritization of this issue (high enough on the agenda to make a public fuss about it).
This seems like a potentially useful term/concept, but I hadn't come across it before and there are only 1890 hits on google for "compliance trace", and none of the hits on the first page seem relevant. Is this a term you're coining here? Is it a translation of an established term from another language?
(I ask because I had made myself an Anki card to remember the term so I could use it later, but then googled to check your definition was ~the standard one and found that maybe it's not an established term so people might be confused if I used it.
It is not coming from another language, I just wanted to broaden the concept of "audit trail" (which is much more established) to encompass all of compliance-related items. I also didn't want to imply it was as sequential/neat as a trail, so I went for trace. I am not a native English speaker so perhaps "trace" is not the right term.