Summary: I model the contribution to AI safety by academics working in adjacent areas. I argue that this contribution is at least on the same order as the EA bet, and seek a lower bound. Guesstimates here and here. I focus on present levels of academic work, but the trend is even more important.
Confidence: High in a notable contribution, low in the particular estimates. Lots of Fermi estimates.
A big reason for the EA focus on AI safety is its neglectedness:
...less than $50 million per year is devoted to the field of AI safety or work specifically targeting global catastrophic biorisks.
80,000 Hours (2019)
...we estimate fewer than 100 people in the world are working on how to make AI safe.
80,000 Hours (2017)
Grand total: $9.09m… [Footnote: this] doesn’t include anyone generally working on verification/control, auditing, transparency, etc. for other reasons.
Seb Farquhar (2018)
...what we are doing is less than a pittance. You go to some random city... Along the highway you see all these huge buildings for companies... Maybe they are designing a new publicity campaign for a razor blade. You drive past hundreds of these... Any one of those has more resources than the total that humanity is spending on [AI safety].
Nick Bostrom (2016)
Numbers like these helped convince me that AI safety is the best thing to work on. I now think that these are underestimates, because of non-EA lines of research which weren't counted.
Use "EA safety" for the whole umbrella of work done at organisations like FHI, MIRI, DeepMind and OpenAI’s safety teams, and by independent researchers. A lot of this - maybe a third - is conducted at universities; to avoid double counting I count it as EA and not academia.
The argument:
- EA safety is small, even relative to a single academic subfield.
- There is overlap between capabilities and short-term safety work.
- There is overlap between short-term safety work and long-term safety work.
- So AI safety is less neglected than the opening quotes imply.
- Also, on present trends, there’s a good chance that academia will do more safety over time, eventually dwarfing the contribution of EA.
What’s ‘safety’?
EA safety is best read as about “AGI alignment”: work on assuring that the actions of an extremely advanced system are sufficiently close to human-friendly goals.
EA focusses on AGI because weaker AI systems aren’t thought to be directly tied to existential risk. However, Critch and Krueger note that “prepotent” - unstoppably advanced, but not necessarily human-level - AI could still pose x-risks. The potential for this latter type is key to the argument that short-term work is relevant to us, since the scaling curves for some systems seem to be holding up, and so might reach prepotence.
“ML safety” could mean just making existing systems safe, or using existing systems as a proxy for aligning an AGI. The latter is sometimes called “mid-term safety”, and this is the key class of work for my purposes.
In the following “AI safety” means anything which helps us solve the AGI control problem.
De facto AI safety work
The line between safety work and capabilities work is sometimes blurred. A classic example is ‘robustness’: it is both a safety problem and a capabilities problem if your system can be reliably broken by noise. Transparency (increasing direct human access to the goals and properties of learned systems) is the most obvious case of work relevant to capabilities, short-term safety, and AGI alignment. As well as being a huge academic fad, it's a core mechanism in 6 out of the 11 live AGI alignment proposals recently summarised by Hubinger.
More controversial is whether there’s significant overlap between short-term safety and AGI alignment. All we need for now is:
The mid-term safety hypothesis (weak form): at least some work on current systems will transfer to AGI alignment.
Some researchers who seem to put a lot of stock in this view: Shah, Christiano, Krakovna, Olsson, Olah, Steinhardt, Amodei, Krueger. (Note that I haven’t polled them; this is guessed from public statements and revealed preferences.) Since 2016, actually “about half” of MIRI’s research has been on their ML agenda, apparently to cover the chance of prosaic AGI.
Here are some alignment-relevant research areas dominated by non-EAs. I won’t explain these: I use the incredibly detailed taxonomy (and 30 literature reviews) of Critch and Krueger (2020). Look there, and at related agendas for explanations and bibliographies.
- Transparency
- Robustness
- Interactive AI
- Calibration
- Formal verification
- Preference learning
- Modelling human cognition
- Safe handovers (AKA corrigibility)
- Assured Autonomy
- Open source game theory
- Multi-agent coordination
- Emergent communication
- Safe RL
- (Parts of) algorithmic fairness
These are narrowly drawn from ML, robotics, and game theory: this is just a sample of relevant work! Work in social science, moral uncertainty, or decision theory could be just as relevant as the above direct technical work; Richard Ngo lists many questions for non-AI people here.
Work in these fields could help directly, if the eventual AGI paradigm is not too dissimilar from the current one (that is, if the weak mid-term hypothesis holds). But there are also indirect benefits: if they help us to use AIs to align AGI; if they help to build the field; if they help convince people that there really is an AGI control problem (for instance, Victoria Krakovna’s specification gaming list has been helpful to me in interacting with sceptical specialists). These imply another view under which much academic work has alignment value:
The mid-term safety hypothesis (very weak form): at least some work on current systems will probably help with AGI alignment in some way, not limited to direct technical transfer.
A natural objection is that most of the above areas don’t address the AGI case: they’re not even trying to solve our problem. I discuss this and other discounts below.
Current levels of safety-related work
How large is EA Safety?
Some overlapping lists:
- # people with posts on the Alignment Forum since late 2018: 94. To my knowledge, 37 of these are full-time.
- 80k AI Safety Google Group: 400, almost entirely junior people.
- Larks’ great 2019 roundup contained ~110 AI researchers (who published that year), most of whom could be described as EA or adjacent.
- Issa Rice’s AI Watch: “778” (raw count, but there’s lots of false positives for general x-risk people and inactive people. Last big update 2018).
In the top-down model I start with all EAs and then filter them by interest in AI risk, direct work, and % of time working on safety. (EA safety has a lot of hobbyists.) The bottom-up model attempts a headcount.
How large is non-EA Safety?
A rough top-down point estimate of academic computer scientists alone working on AI gives 84k to 103k, with caveats summarised in the Guesstimate. Then define a (very) rough relevance filter:
C = % of AI work on capabilities
S = % of AI work on short-term safety
CS = % of capabilities work that overlaps with short-term safety
SL = % of short-term safety that overlaps with long-term safety
Then, we could decompose the safety-relevant part of academic AI as:
SR = (C * CS * SL) + (S * SL)
Then the non-EA safety size is simply the field size * SR.
None of those parameters is obvious, but I make an attempt in the model (bottom-left corner).
This just counts academia, and just technical AI within that. It's harder to estimate the amount of industrial effort, but the AI Index report suggests that commercial AI research is about 10% as large as academic research (by number of papers, not impact). But we don't need this if we're just arguing that the non-EA lower bound is large.
What’s a good discount factor for de facto safety work?
In EA safety, it’s common to be cynical about academia and empirical AI safety. There’s something to it: the amount of paperwork and communication overhead is notorious; there are perverse incentives around publishing tempo, short-termism, and conformity; it is very common to emphasise only the positive effects of your work; and, as the GPT-2 story shows, there is a strong dogma about automatic disclosure of all work. Also, insofar as AI safety is ‘pre-paradigmatic’, you might not expect normal science to make much headway. (But note that several agent-foundation-style models are from academia - see ‘A cursory check’ below.)
But this is only half of the ledger. One of the big advantages of academic work is the much better distribution of senior researchers: EA Safety seems bottlenecked on people able to guide and train juniors. Another factor is increased influence: the average academic has serious opportunities to affect policy, hundreds of students, and the general attitude of their field toward alignment, including non-academic work on alignment. Lastly, you get access to government-scale funding. I ignore these positives in the following.
Model
Here’s a top-down model arguing that technical AI academics could have the same order of effect as EA, even under a heavy impact discount, even when ignoring other fields and the useful features of academia. Here’s an (incomplete) bottom-up model to check if it’s roughly sensible. As you can see from the variance, the output means are not to be trusted.
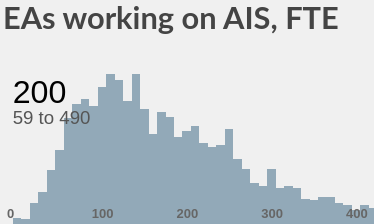

A “confidence” interval
Again, the model is conservative: I don’t count the most prominent safety-relevant academic institutions (FHI, CHAI, etc); I don’t count contributions from industry, just the single most relevant academic field; I don’t count non-technical academic contributions; and a high discount is applied to academic work. For the sake of argument I’ve set the discount very high: a unit of adjacent academic work is said to be 80% less effective than a unit of explicit AGI work. The models rely on my priors; customise them before drawing conclusions (see ‘Parameters’ below).
A cursory check of the model
The above implies that there should be a lot of mainstream work with alignment implications - maybe as much as EA produces. A systematic study would be a big undertaking, but can we at least find examples? Yes:
- AIXI (2000), a theoretically optimal RL agent.
- Gödel machines (2003), the limit case of verified self-improvement.
- Inverse reinforcement learning (2004 - 2016). A limited but fruitful model for thinking about value learning.
- Various forms of Imitation learning
- Active learning, particularly TAMER (2009) and active reward learning (2014).
- Info-theoretic measures of control like empowerment (2005).
- Adversarial training (2015). As used in AI Safety Debate.
- Wooldridge on the game-theoretic / social choice agent foundations of AI.
- Existence proof for the short/long-term overlap: The Stanford “Center for AI Safety” is a good example of such work. Zero mention of AGI or alignment while working on many of the de facto topics.
How much does EA safety produce? In Larks’ exhaustive annual round-up of EA safety work in 2019, he identified about 50 paper-sized chunks (not counting MIRI’s private efforts). Of them, both CAIS and mesa-optimisers seem more significant than the above. Recent years have seen similarly important EA work (e.g. Debate, quantilizers, or the Armstrong/Shah discussion of value learning).
What does this change?
I argue that AIS is less neglected than it seems, because some academic work is related, and academia is enormous. (My confidence interval for the academic contribution is vast - but I didn’t quite manage to zero out the lower bound even by being conservative.) Does this change the cause’s priority?
Probably not. Even if the field is bigger than we thought, it’s still extremely small relative to the investment in AI capabilities, and highly neglected relative to its importance. The point of the above is to correct your model, to draw attention to other sources of useful work, and to help sharpen a persistent disagreement within EA safety about the role of mid-term safety and academia.
This might change your view of effective interventions within AIS (for instance, ways to bring AGI alignment further within the Overton window), but my model doesn’t get you there on its own. A key quantity I don’t really discuss is the ratio of capabilities to alignment work. It seems prohibitively hard to reduce capabilities investment. But a large, credible academic field of alignment is one way to replace some work on capabilities.
Future safety-related work
A naive extrapolation implies that AIS neglectedness will decrease further: in the last 10 years, Safety has moved from the fringe of the internet into the heart of great universities and NGOs. We have momentum: the programme is supported by some of the most influential AI researchers - e.g. Russell, Bengio, Sutskever, Shanahan, Rossi, Selman, McAllester, Pearl, Schmidhuber, Horvitz. (Often only verbal approval.)
In addition, from personal experience, junior academics are much more favourable towards alignment and are much more likely to want to work on it directly.
Lastly: Intuitively, the economic incentive to solve AGI-safety-like problems scales as capabilities increase, and as mid-term problems draw attention. Ordinary legal liability disincentivises all the sub-existential risks. (The incentive may not scale properly, from a longtermist perspective, but the direction still seems helpful.)
If this continues, then even the EA bet on direct AGI alignment could be totally outstripped by normal academic incentives (prestige, social proof, herding around the agendas of top researchers).
A cool forecasting competition is currently running on a related question.
This argument depends on our luck holding, and moreover, on people (e.g. me) not naively announcing victory and so discouraging investment. But to the extent that you trust the trend, this should affect your prioritisation of AI safety, since its expected neglectedness is a great deal smaller.
Parameters
- Your probability of prosaic AGI (i.e. where we get there by just scaling up black-box algorithms). Whether it’s possible to align prosaic AGI. Your probability that agent foundations is the only way to promote real alignment.
- The percentage of mainstream work which is relevant to AGI alignment. Subsumes the capabilities/safety overlap and the short/long term safety overlap. The idea of a continuous discount on work adjacent to alignment would be misguided if there were really two classes of safety problem, short- and long-term, and if short-term work had negligible impact on the long-term problems. The relevance would then be near 0.
- The above is extremely sensitive to your forecast for AGI. Given very short timelines, you should focus on other things than climbing up through academia, even if you think it’s generally well-suited to this task; conversely, if you think we have 100 years, then you can have pretty strong views on academic inadequacy and still agree that their impact will be substantial.
- If you have an extremely negative view of academia's efficiency, then the above may not move you much. (See for instance, the dramatically diminishing return on inputs in mature fields like physics.)
Caveats, future work
- To estimate academia fairly, you’d need a more complicated model, involving second-order effects like availability of senior researchers, policy influence, opportunity to spread ideas to students and colleagues, funding. That is, academia has extremely clear paths to global impact. But since academia is stronger on the second order, omitting it doesn’t hurt my lower-bound argument.
- A question which deserves a post of its own is: “How often do scientists inadvertently solve a problem?” (The general form - “how often does seemingly unrelated work help? Provide crucial help?” - seems trivial: many solutions are helped by seemingly unrelated prior work.) I’m relying on the overlap parameters to cover the effect of “actually trying to solve the problem", but this might not be apt. Maybe average academia is to research as the average charity is to impact: maybe directly targeting impact is that important.
- I haven’t thought much about potential harms from academic alignment work. Short-termists crowding out long-termists and a lack of attention to info hazards might be two.
- Intellectual impact is not linear in people. Also, the above treats all (non-EA) academic institutions as equally conducive to safety work, which is not true.
- Even more caveats.
- Conflict of interest: I’m a PhD student.
Thanks to Jan Brauner for the idea. Thanks to Vojta Kovařík, Aaron Gertler, Ago Lajko, Tomáš Gavenčiak, Misha Yagudin, Rob Kirk, Matthijs Maas, Nandi Schoots, and Richard Ngo for helpful comments.
Thanks for writing this post--it was useful to see the argument written out so I could see exactly where I agreed and disagreed. I think lots of people agree with this but I've never seen it written up clearly before.
I think I place substantial weight (30% or something) on you being roughly right about the relative contributions of EA safety and non-EA safety. But I think it's more likely that the penalty on non-EA safety work is larger than you think.
I think the crux here is that I think AI alignment probably requires really focused attention, and research done by people who are trying to do something else will probably end up not being very helpful for some of the core problems.
It's a little hard to evaluate the counterfactuals here, but I'd much rather have the contributions from EA safety than from non EA safety over the last ten years.
I think that it might be easier to assign a value to the discount factor by assessing the total contributions of EA safety and non-EA safety. I think that EA safety does something like 70% of the value-weighted work, which suggests a much bigger discount factor than 80%.
---
Assorted minor comments:
Yes, but those senior researchers won't necessarily have useful things to say about how to do safety research. (In fact, my impression is that most people doing safety research in academia have advisors who don't have very smart thoughts on long term AI alignment.)
I think the link is to the wrong model?
In this section you count nine safety-relevant things done by academia over two decades, and then note that there were two things from within EA safety last year that seem more important. This doesn't seem to mesh with your claim about their relative productivity.
Was going to write a longer comment but I basically agree with Buck's take here.
I wanted to endorse this in particular.
On the actual argument:
I agree with 1, 2, and 3 (though perhaps disagree with the magnitude of 2 and 3, e.g. you list a bunch of related areas and for most of them I'd be surprised if they mattered much for AGI alignment).
I agree 4 is literally true, but I'm not sure it necessarily matters, as this sort of thing can be said for ~any field (as Ben Todd notes). It would be weird to say that animal welfare is not neglected because of the huge field of academia studying animals, even though those fields are relevant to questions of e.g. sentience or farmed animal welfare.
I strongly agree with 5 (if we replace "academia" with "academia + industry", it's plausible to me academia never gets involved while industry does), and when I argue that "work will be done by non-EAs", I'm talking about future work, not current work.
Thanks!
Yeah, it'd be good to break AGI control down more, to see if there are classes of problem where we should expect indirect work to be much less useful. But this particular model already has enough degrees of freedom to make me nervous.
That would be great! I used headcount because it's relatively easy, but value weights are clearly better. Do you know any reviews of alignment contributions?
Yeah, I don't claim to be systematic. The nine are just notable things I happened across, rather than an exhaustive list of academic contributions. Besides the weak evidence from the model, my optimism about there being many other academic contributions is based on my own shallow knowledge of AI: "if even I could come up with 9..."
Something like the Median insights collection, but for alignment, would be amazing, but I didn't have time.
This might be another crux: "how much do general AI research skills transfer to alignment research?" (Tacitly I was assuming medium-high transfer.)
No, that's the one; I mean the 2x2 of factors which lead to '% work that is alignment relevant'. (Annoyingly, Guesstimate hides the dependencies by default; try View > Visible)
My intuition is also that the discount for academia solving core alignment problems should be (much?) higher than here. At the same time I agree that some mainstream work (esp. foundations) does help current AI alignment research significantly. I would expect (and hope) more of this to still appear, but to be increasingly sparse (relative to amount of work in AI).
I think that it would be useful to have a contribution model that can distinguish (at least) between a) improving the wider area (including e.g. fundamental models, general tools, best practices, learnability) and b) working on the problem itself. Distinguishing past contribution and expected future contribution (resp. discount factor) may also help.
Why: Having a well developed field is a big help in solving any particular problem X adjacent to it and it seems reasonable to assign a part of the value of "X is solved" to work done on the field. However, field development alone is unlikely to solve X for sufficiently hard X that is not in the field's foci, and dedicated work on X is still needed. I imagine this applies to the field of ML/AI and long-termist AI alignment.
Model sketch: General work done on the field has diminishing returns towards the work remaining on the problem. As the field grows, it branches and the surface area grows as a function of this, and the progress in directions that are not foci slows appropriately. Extensive investment in the field would solve any problem eventually but unfocused effort would is increasingly inefficient. Main uncertainties: I am not sure how to model areas of field focus and the faster progress in their vicinity, or how much I would expect some direction sufficiently close to AI alignment to be a focus of AI.
Overall, this would make me to expect that the past work in AI and ML would have a significant contribution towards AI alignment but to expect increasing discount in the future, unless alignment becomes a focus for AI or close to it. When thinking about policy implications for focusing research effort (with the goal of solving AI alignment), I would expect the returns to general academia to diminish much faster than to EA safety research.
Considering the research necessary to "solve alignment for the AIs that will actually be built" as some nodes in the directed acyclic graph of scientific and engineering progress, another crux seems to me to be how effective it is to do that research with the input nodes available today to an org focused specifically on AI alignment:
My intuition there is that progress on fundamental, mathematically hard or even philosophical questions is likely to come serendipitously from people with academic freedom, who happen to have some relevant input nodes in their head. On the other hand, for an actual huge Manhattan-like engineering project to build GAI, making it safe might be a large sub-project itself - but only the engineers involved can understand what needs to be done to do so, just like the Wright brothers wouldn't have much to say about making a modern jet plane safe.
Hi there, thanks for the post - useful figures!
I agree with the central point, though I want to point out this issue applies to most of the problem areas we focus on. This means it would only cause you to down-rate AI safety relative to other issues if you think the 'spillover' from other work is greater for AI safety than for other issues.
This effect should be bigger for causes that appear very small, so it probably does cause AI safety to look less neglected relative to, say, climate change, but maybe not relative to global priorities research. And in general, these effects mean that super neglected causes are not as good as they first seem.
That said, it's useful to try to directly estimate the indirect resources for different issues in order to check this, so I'm glad to have these specific estimates.
There is some more discussion of this general issue in our problem framework article:
If the above strikes you as wrong (and not just vague), you could copy the Guesstimate, edit the parameters, and comment below.
[Epistemic status: third-hand information, definitely check with someone from MIRI. ETA: confirmed by MIRI's Rob Bensinger below.]
My impression is that MIRI never did much work on the ML agenda you link to because the relevant key researchers left.
For a few years, they do seem to have been doing a lot of nonpublic work that's distinct from their agent foundations work. But I don't know how related that work is to their old ML agenda, and what fraction of their research it represents.
I agree with Max's take. MIRI researchers still look at Alignment for Advanced Machine Learning Systems (AAMLS) problems periodically, but per Why I am not currently working on the AAMLS agenda, they mostly haven't felt the problems are tractable enough right now to warrant a heavy focus.
Nate describes our new work here: 2018 Update: Our New Research Directions.
I don't think any of MIRI's major research programs, including AAMLS, have been focused on prosaic AI alignment. (I'd be interested to hear if Jessica or others disagree with me.)
Paul introduced prosaic AI alignment (in November 2016) with:
In contrast, I think of AAMLS as assuming that we'll need new deep insights into intelligence in order to actually align an AGI system. There's a large gulf between (1) "Prosaic AGI alignment is feasible" and (2) "AGI may be produced by techniques that are descended from current ML techniques" or (3) "Working with ML concepts and systems can help improve our understanding of AGI alignment", and I think of AAMLS as assuming some combination of 2 and 3, but not 1. From a post I wrote in July 2016:
Indeed, Why I am not currently working on the AAMLS agenda is a year-later write up by the lead researcher. Moreover, they write:
Thanks for this info.
Just a heads up that the link seems to lead back to this post. I think you mean to link to this?
Thanks; fixed.
MIRI is not optimistic about prosaic AGI alignment and doesn't put much time into it.
On the other hand, in 2018's review MIRI wrote about new research directions, one of which feels ML adjacent. But from a few paragraphs, it doesn't seem that the direction is relevant for prosaic AI alignment.
Thanks for this, I've flagged this in the main text. Should've paid more attention to my confusion on reading their old announcement!
If you want some more examples of specific research/researchers, a bunch of the grantees from FLI's 2015 AI Safety RFP are non-EA academics who have done some research in fields potentially relevant to mid-term safety.
https://futureoflife.org/ai-safety-research/
I would like to highlight an aspect you mention in the "other caveats": How much should you discount for Goodharting vs doing things for the right reasons? Or, relatedly, if you work on some relevant topic (say, Embedded Agency) without knowing that AI X-risk could be a thing, how much less useful will your work be? I am very uncertain about the size of this effect - maybe it is merely a 10% decrease in impact, but I wouldn't be too surprised if it decreased the amount of useful work by 98% either.
Personally, I view this as the main potential argument against the usefulness of academia. However, even if the effect is large, the implication is not that we should ignore academia. Rather, it would suggest that we can get huge gains by increasing the degree to which academics do the research because of the right reasons.
(Standard disclaimers apply: This can be done in various ways. Viktoria Krakovna's list of specification gaming examples is a good one. Screaming about how everybody is going to die tomorrow isn't :P.)
Vojta
This post is really approximate and lightly sketched, but at least it says so. Overall I think the numbers are wrong and the argument is sound.
Synthesising responses:
Industry is going to be a bigger player in safety, just as it's a bigger player in capabilities.
My model could be extremely useful if anyone could replace the headcount with any proxy of productivity on the real problem. Any proxy at all.
Doing the bottom up model was one of the most informative parts for me. You can cradle the whole field in the palm of your mind. It is a small and precious thing. At the same time, many organisations you would assume are doing lots of AGI existential risk reduction, are not.
Half of the juice of this post is in the caveats and the caveat overflow gdoc.
I continue to be confused by how little attention people pay to the agendas, in particular the lovely friendly CHAI bibliography.
Todd notes that you could say the same about most causes, everything is in fact connected. If this degree of indirect effect was uniform, then the ranking of causes would be unchanged. But there's something very important about the absolute level, and not just for timelines and replaceability. Safety people need gears, and "is a giant wave of thousands of smart people going to come?" is a big gear.
A lot can change in 3 years, at MIRI.
An important source of capabilities / safety overlap, via Ben Garfinkel:
He sees this as positive: it implies massive economic incentives to do some alignment, and a block on capabilities until it's done. But it could be a liability as well, if the alignment of weak systems is correspondingly weak, and if mid-term safety work fed into a capabilities feedback loop with greater amplification.
Important question, and nicely researched!
A caveat is that some essential subareas of safety may be neglected. This is not a problem when subareas substitute each other: e.g. debate substitutes for amplification so it's okay if one of them is neglected. But there's a problem when subareas complement each other: e.g. alignment complements robustness so we probably need to solve both. See also When causes multiply.
It's ok when a subarea is neglected as long as there's a substitute for it. But so far it seems that some areas are necessary components of AI safety (perhaps both inner and outer alignment are).
This makes sense. I don't mean to imply that we don't need direct work.
AI strategy people have thought a lot about the capabilities : safety ratio, but it'd be interesting to think about the ratio of complementary parts of safety you mention. Ben Garfinkel notes that e.g. reward engineering work (by alignment researchers) is dual-use; it's not hard to imagine scenarios where lots of progress in reward engineering without corresponding progress in inner alignment could hurt us.
This is a very important subject. I've also come to a similar conclusion independently recently -- that a lot of the AI Safety work being funded by EA orgs (OpenPhil in particular sticks out) is prosaic AI alignment work that is not very neglected. Perversely, as the number of academic and commercial researchers and practitioners working on prosaic alignment as exploded, there has been a shift in the AI Safety community towards prosaic AI work and away from agent foundations type work. From what I hear, that has mainly been because of concerns that scaling transformer-like models will lead to existentially dangerous AI, which I personally think is misguided for reasons that would take too much space to go into here. [I personally think scaling up transformers / deep learning will be part of the AGI puzzle but there are missing pieces, and I would rather people try to figure out what those missing pieces are than focus so much on safety and alignment with deep learning which is not neglected at this point.]
I think this post missed how much prosaic AI safety work is being in commercial settings, since it only focused on academia. I am currently working to deploy models from both commercial companies and academic research labs into hospital radiology clinics so we can gather real world data on performance and get feedback from radiologists. The issue of lack of robustness / distribution shift is a huge problem and we are having to develop additional AI models to identify outliers and anomalies and do automated quality control on images to make sure the right body part is shown and its in the right orientation for whatever AI model we are trying to run. I would argue that every major company trying to deploy ML/DL is struggling with similar robustness issues and there is enormous commercial/market incentives to solves such issues. Furthermore, there is intense pressure on companies to make their models explainable (see the EU's GDPR legislation which gives users a "right to an explanation" in certain cases). We also see this with transformers - after various debacles at Google (like the black people=Gorillas case in Google photos, anti-semitic autocompletes, etc) and Microsoft (Tay), I'm pretty confident both companies will be putting a lot of effort into aligning transformers (the current default for transformer models is to spew racist crap and misinformation at random intervals which is a complete no-go from a commercial perspective).
So, a lot (but not all!) of the work going on at Anthropic and Redwood, to be perfectly frank, seems very un-neglected to me, although there is an argument that academics will have trouble doing such work because of problems accessing compute and data. [To be clear, I still think its worth having EAs doing alignment with the AI methods/models at the frontier of various benchmarks with an eye to where thinks are going in the future, but what I take issue with is the relative amount of EA funding flowing into such work right now. ]
The amount of research going on in both industry and academia under the umbrella of "fairness, accountability, and transparency" has exploded recently (see the ACM FAccT conference). Research on explainability methods has exploded too (a very non-exhaustive list I did in early 2020 includes some 30 or so explainability methods and I've seen graphs showing that the number of papers on explainability /interpretability/XAI is growing exponentially).
There is a very important point I want to make on explainability work in particular though - most of it is "post-hoc" explainability where the main goal is increasing trust (which often, to be frank, just involves showing pretty pictures and telling stories and not actually empirically measuring if trust is increased and if the increased trust is warranted/good). (Sometimes researchers also want to debug failures and detect bias, also, although I have not found many non-trivial cases where this has been achieved). I believe 90%+ percent of current explainability work has very little value to actually understanding how AI models work mechanistically and thus has no relevance to AI existential safety. I keep pointing people to the work of Adebayo et al showing that the most popular XAI methods today are useless for actual mechanistic understanding and the work of Hase et al. showing popular XAI methods don't help humans much to predict how an ML model will behave. So, work on tools and methods for generating and validating mechanistic explanations in particular still seems very neglected. The only real work that goes to the "gears level" (ie level of neurons/features and weights/dependencies) is the "Circuits" work by Olah et al. at OpenAI. The issue with mechanistic explainability work though is the high amount of work/effort required and that it may be hard to transfer methods to future architectures. For instance if AI moves from using transformers to something like Hebbian networks, energy based models, spiking neural nets, or architectures designed by genetic algorithms (all plausible scenarios in my view) then entirely new tool chains will have to be built and plausibly some methods will have to be thrown out completely and entirely new ones developed. Of course, this is all very unclear... I think more clarity will be obtained once we see how well the tools and methods developed by Olah et al. for CNNs transfer to transformers, which I hear is something they are doing at Anthropic.
Thanks Dan. I agree that industry is more significant (despite the part of it which publishes being ~10x smaller than academic AI research). If you have some insight into the size and quality of the non-publishing part, that would be useful.
Do language models default to racism? As I understand the Tay case, it took thousands of adversarial messages to make it racist.
Agree that the elision of trust and trustworthiness is very toxic. I tend to ignore XAI.
Follow-up post to ARCHES with ranking of existing fields, lots more bibliographies.
This post annoyed me. Which is a good thing! It means that you hit where it hurts, and you forced me to reconsider my arguments. I also had to update (a bit) toward your position, because I realized that my "counter-arguments" weren't that strong.
Still, here they are:
With all that being said, I'm glad you wrote this post and I think I'll revisit it and think more about it.
Could you say more (or work on that post) about why formal methods will be unhelpful? Why are places like Stanford, CMU, etc. pushing to integrate formal methods with AI safety? Also Paul Christiano has suggested formal methods will be useful for avoiding catastrophic scenarios. (Will update with links if you want.)
Hum, I think I wrote my point badly on the comment above. What I mean isn't that formal methods will never be useful, just that they're not really useful yet, and will require more pure AI safety research to be useful.
The general reason is that all formal methods try to show that a program follows a specification on a model of computation. Right now, a lot of the work on formal methods applied to AI focus on adapting known formal methods to the specific programs (say Neural Networks) and the right model of computation (in what contexts do you use these programs, how can you abstract their execution to make it simpler). But one point they fail to address is the question of the specification.
Note that when I say specification, I mean a formal specification. In practice, it's usually a modal logic formula, in LTL for example. And here we get at the crux of my argument: nobody knows the specification for almost all AI properties we care about. Nobody knows the specification for "Recognizing kittens" or "Answering correctly a question in English". And even for safety questions, we don't have yet a specification of "doesn't manipulate us" or "is aligned". That's the work that still needs to be done, and that's what people like Paul Christiano and Evan Hubinger, among others, are doing. But until we have such properties, the formal methods will not be really useful to either AI capability or AI safety.
Lastly, I want to point out that working on AI for formal methods is also a means to get money and prestige. I'm not going to go full Hanson and say that's the only reason, but it's still a part of the international situation. I have examples of people getting AI related funding in France, for a project that is really, but really useless for AI.
Really interesting and informative - thanks for writing this!
On midterm safety: I think this is underexplored and not discussed enough here.
I have been thinking that generating more examples of how midterm safety risks could be catastrophic might be helpful (e.g. fusion power is apparently bottlenecked by computing power and algorithmic advances). Btw I thought this video by Stuart Armstrong on specification gaming was really good for skeptical specialists.
Also, I feel like more writing on midterm safety could be combined with Greg Lewis' post on GCRs importance from a person affecting view because that significantly relaxes assumptions about the importance of AI safety that might seem alien to skeptics.